Artificial Intelligence in Pharmaceutical Technology and Drug Delivery Design

Abstract
Artificial intelligence (AI) has emerged as a powerful tool that harnesses anthropomorphic knowledge and provides expedited solutions to complex challenges. Remarkable advancements in AI technology and machine learning present a transformative opportunity in the drug discovery, formulation, and testing of pharmaceutical dosage forms. By utilizing AI algorithms that analyze extensive biological data, including genomics and proteomics, researchers can identify disease-associated targets and predict their interactions with potential drug candidates. This enables a more efficient and targeted approach to drug discovery, thereby increasing the likelihood of successful drug approvals.
Furthermore, AI can contribute to reducing development costs by optimizing research and development processes. Machine learning algorithms assist in experimental design and can predict the pharmacokinetics and toxicity of drug candidates. This capability enables the prioritization and optimization of lead compounds, reducing the need for extensive and costly animal testing. Personalized medicine approaches can be facilitated through AI algorithms that analyze real-world patient data, leading to more effective treatment outcomes and improved patient adherence. This comprehensive review explores the wide-ranging applications of AI in drug discovery, drug delivery dosage form designs, process optimization, testing, and pharmacokinetics/pharmacodynamics (PK/PD) studies.
This review provides an overview of various AI-based approaches utilized in pharmaceutical technology, highlighting their benefits and drawbacks. Nevertheless, the continued investment in and exploration of AI in the pharmaceutical industry offer exciting prospects for enhancing drug development processes and patient care.
Introduction
Numerous industries are striving to enhance their progress to meet the demands and expectations of their customers, utilizing various methodologies. The pharmaceutical industry is a critical field that plays a vital role in saving lives. It operates based on continuous innovation and the adoption of new technologies to address global healthcare challenges and respond to medical emergencies, such as the recent pandemic [1]. In the pharmaceutical industry, innovation is typically predicated on extensive research and development across various domains, including but not limited to manufacturing technology, packaging considerations, and customer-oriented marketing strategies [2]. Novel pharmaceutical innovations are range from small drug molecules to biologics, with a preference for better stability with high potency to fulfil unmet needs to treat diseases. The assessment of the significant levels of toxicity associated with new drugs is an area of considerable concern, necessitating extensive research and exploration in the foreseeable future. One of the primary aims is to provide drug molecules that offer optimal benefits and suitability for utilization in the healthcare industry. Despite this, the pharmacy industry faces numerous obstacles that necessitate further advancement using technology-driven methods to address worldwide medical and healthcare demands [3,4,5].
The need for a proficient workforce in the healthcare industry is persistent, necessitating the continuous provision of training to healthcare personnel to augment their involvement in routine duties. Identifying skill gaps in the workplace is a crucial undertaking within the pharmaceutical industry. It is imperative to effectively address the identified gaps through appropriate remedial measures while acknowledging that providing adequate training can also pose a significant challenge. As per a report presented by certain authorities, it has been observed that approximately 41% of supply chain disruptions occurred in June 2022. The report further highlights that supply chain disruption has emerged as the second-most-formidable challenge to overcome. Several pharmaceutical industries are anticipating further advancements in their supply chain, as well as innovative models to address these challenges, with the potential to enhance business resilience [6]. The global outbreak of coronavirus disease 2019 (COVID-19) has caused significant disruptions to various operations worldwide, including ongoing clinical trials [7].
Pandemics, natural catastrophes, pricing changes, cyberattacks, logistical delays, and product issues increase supply chain disruptions. Transportation challenges caused by the epidemic have devastated the supply chain network and global industries. Decision-induced delays for price updates from suppliers owing to misunderstanding over whether to utilize the new price or the existing price for commodities or materials create price fluctuation delays. New obstacles arise from countries’ cross-border trade cooperation strategies, increasing criminal activity and instability in the availability of crucial resources for operation and production. The manufacturing of footprint modifications is needed to suit patient needs and compliance.
Within the pharmaceutical industry, a significant quantity of COVID-19 vaccines ended up being unusable during the pandemic because of complications related to the maintenance of the cold chain. The primary cause of supply chain disruption resulting from the delayed response can be attributed to insufficient innovation and imprecise forecasting in industrial and commercial operations. Supply chain disruptions within the pharmaceutical industry have significant ramifications on customer satisfaction, corporate reputation, and potential profits [8,9].
The implementation of AI is poised to bring about a significant transformation in the way the pharmaceutical industry handles supply chain operations (Figure 1). It also consolidates numerous AI research endeavors from recent decades to create effective solutions for diverse supply chain issues. Additionally, the study suggests potential research areas that could enhance decision-making tools for supply chain management in the future [10,11].
Table 1. Top 10 list of commonly used AI models in the pharmaceutical industry.
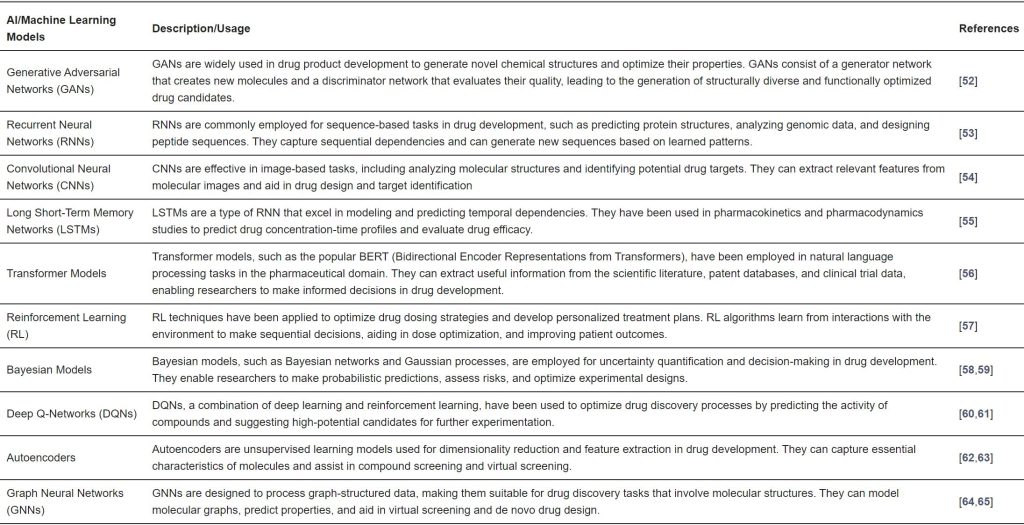
The primary impact of the pandemic is receding, but it still has some influence on clinical trials. Many pharmaceutical companies are looking to adopt newer technologies, including platforms such as AI and virtual platforms in this field. These new technologies may be helpful in the restart or recreation of these clinical trials, with minimal interaction for face-to-face types [12,13,14,15,16,17,18], as presented in Figure 1. At present, highly skilled workers and high maintenance costs pose a larger challenge. The fourth main challenge in seeking a technology-based solution is data breaches and cybersecurity threats. The number of cyberattacks on available patient data has also increased in the 21st century, and many pharmaceutical companies are more concerned about confidential medical records and patient data, which are especially vulnerable to cybersecurity attacks. Some of the major challenges associated with traditional clinical trials are data fragmentation and disconnected system involvement, which generally result from scattered data generated during the trials and hence require extensive manual data transcription efforts for documents along with those of the systems. There is a lack of innovation in the trial models, which thus requires the rework and repetition of the ongoing work. In the healthcare sector, patient recruitment, enrollment, monitoring, retention, and medical adherence are the key points that require special attention due to clinical trials. The enrollment of the patient is affected due to the traveling process at the trial sites, which is time-consuming for the participants, and frequent visits to sites contribute to patient re-enrollment in the same context. The application of AI to the study design helps with optimization as well as accretion for the work related to the creation of the patient-centric type of design. AI uses techniques for the collection of the huge amounts of data generated from those clinical trials, thus reducing the amount of data manpower required for the same. Such technologies implement body sensors along with wearable devices to record the patient’s vital signs and valuable information in a remote mode, which helps meet the patient’s requirement for face-to-face interaction on a routine basis. AI algorithms using wearable technology provide real-time insights during the study process [19].
A new technology platform and solution are required for the implementation of effective cybersecurity inside the office and for remote workers. Special attention must also be paid to data security and breach techniques. Technology is also required to address political fraud, and many cases have been reported, especially during the pandemic in the last few years around the world. Therefore, there is a need to take appropriate steps for the prevention of healthcare fraud, along with constant encouragement for internal discussions about fraudulent behaviors, which may help in the inhibition of the same.
Download the full article as PDF here Artificial Intelligence in Pharmaceutical Technology and Drug Delivery Design
or read it here
Vora, L.K.; Gholap, A.D.; Jetha, K.; Thakur, R.R.S.; Solanki, H.K.; Chavda, V.P. Artificial Intelligence in Pharmaceutical Technology and Drug Delivery Design. Pharmaceutics 2023, 15, 1916. https://doi.org/10.3390/pharmaceutics15071916
Watch our free webinar “Make it Right: Best Practices for mRNA Manufacturing” here:
