Advances in Artificial Intelligence in Drug Delivery and Development: A Comprehensive Review
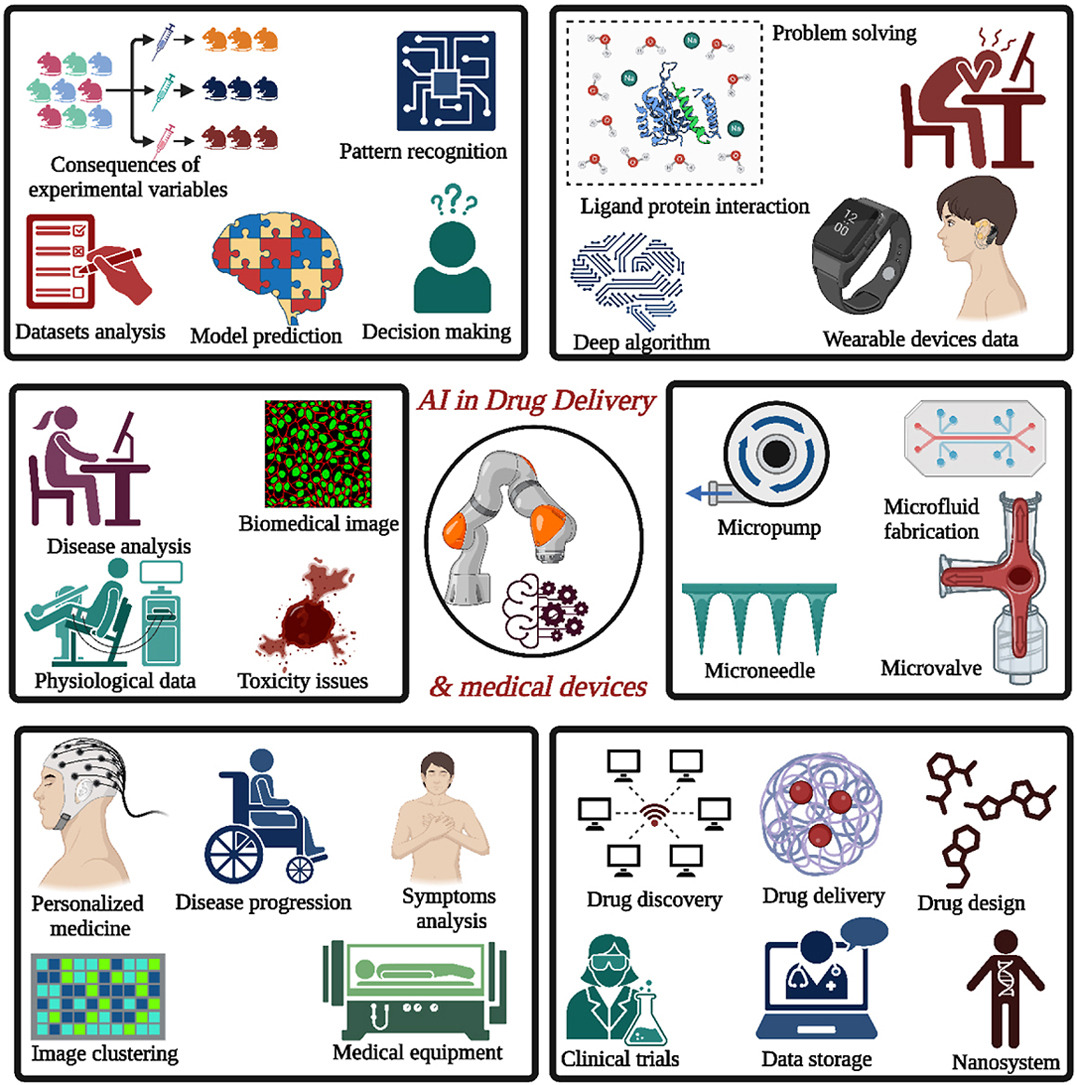
Abstract
Artificial intelligence (AI) has emerged as a powerful tool to revolutionize the healthcare sector, including drug delivery and development. This review explores the current and future applications of AI in the pharmaceutical industry, focusing on drug delivery and development. It covers various aspects such as smart drug delivery networks, sensors, drug repurposing, statistical modeling, and simulation of biotechnological and biological systems. The integration of AI with nanotechnologies and nanomedicines is also examined. AI offers significant advancements in drug discovery by efficiently identifying compounds, validating drug targets, streamlining drug structures, and prioritizing response templates. Techniques like data mining, multitask learning, and high-throughput screening contribute to better drug discovery and development innovations. The review discusses AI applications in drug formulation and delivery, clinical trials, drug safety, and pharmacovigilance. It addresses regulatory considerations and challenges associated with AI in pharmaceuticals, including privacy, data security, and interpretability of AI models. The review concludes with future perspectives, highlighting emerging trends, addressing limitations and biases in AI models, and emphasizing the importance of collaboration and knowledge sharing. It provides a comprehensive overview of AI’s potential to transform the pharmaceutical industry and improve patient care while identifying further research and development areas.
Highlights
- AI revolutionizes healthcare with smart drug delivery and development.
- AI integrates with nanotech for advanced drug discovery.
- Techniques like data mining and high-throughput screening enhance drug innovation.
- AI applications span drug formulation, clinical trials, and pharmacovigilance.
- Challenges include privacy, data security, and model interpretability.
Introduction
Drug delivery technology has evolved remarkably, driven by the need to address ever-increasing challenges. The first generation focused on improving the basic physicochemical properties of drugs, such as solubility, stability, and bioavailability. However, biological barriers like cell membranes and the blood-brain barrier emerged as significant hurdles. This led to the development of second-generation drug delivery systems specifically designed to overcome these biological barriers. These advancements employed mechanisms like enhanced penetration, targeted delivery to specific tissues or cells, and protection from degradation by the body’s defense mechanisms. Ultimately, these second-generation approaches aimed to improve therapeutic efficacy and minimize side effects [1]. Despite the progress made by the second-generation drug delivery technologies, it became clear that a comprehensive approach was needed to address both physicochemical and biological barriers. This led to the development of the third generation of drug delivery technologies. The third-generation drug delivery technologies aim to integrate solutions for both physicochemical and biological challenges. They seek to optimize drug properties, such as solubility, stability, and release kinetics, while also considering strategies to overcome biological barriers and enhance drug delivery to the target site [2]. More recent research has explored various approaches to these interconnected issues. Significant work has focused on improving poor water solubility through formulation strategies like modifying excipients. Developing controlled and targeted delivery of proteins, peptides, and other complex drugs has also been a major focus. The later drug development process increasingly utilizes molecular biology, genomics, recombinant techniques, and monoclonal antibodies to enhance therapeutic profiles. Still, some major challenges must be addressed to effectively overcome human physiology limits, drug intolerance issues, and pathogenic resistance problems demand acceleration of new ways of thinking for possible new methods for better results [3].
Due to the high degree of uncertainty and undiscovered pathophysiology, the complex drug development process also faces challenges in target identification for nervous system issues and disorders. Current pharmaceutical research also faces challenges in recapitulating diseases through animal models addressing patient population heterogeneity through enhanced clinical phenotyping and endotyping [4]. To ensure the proper validation of diagnostic and therapeutic biomarkers for the easy detection of biological states and to navigate the stringent regulatory process, it is crucial to implement appropriate changes at an institutional level to facilitate interdisciplinary explorations. The advancement of technology and the development of skills will play a significant role in addressing these challenges and bridging the gap between academia and the pharmaceutical industry [5]. The industry also faces major challenges, including batch-to-batch variation, ease of process for identification of best drug design, and implementation of economically viable design for profitable business [6]. Innovations are required in various segments of pharmaceutical research to establish robust manufacturing processes that comply with quality specifications, enable ease of continuous manufacturing, minimize process deviations, and ensure compliance with regulatory authorities [7]. These innovations in pharmaceutical manufacturing will help reduce financial and supply risks by enabling effective quality-based production of pharmaceutical products to meet the increasing global demand for medicines [8].
Artificial intelligence (AI) and machine learning (ML) have great potential in drug development, bringing about several improvements in different research sectors. These include the identification of novel targets, a better understanding of disease and target associations, selection of drug candidates, predictions of protein structure, design of molecular compounds, enhanced exploration of disease mechanisms, and progressive research into prognostic and predictive biomarkers. Additionally, AI and ML can provide significant inputs for biometric data analysis through wearable devices, precision medicine, and the execution of clinical trials data analysis for better experimental output in the pandemic era, utilizing proper data collection methods and site monitoring [9].
The rapid advancement of computing capabilities and the refinement of ML and AI has transformed from a theoretical concept to a practical reality, enabling automated tasks performed by machines without human supervision [10]. It exhibits unique and innovative characteristics, showcasing its ability to process information swiftly. Moreover, its cost-effectiveness has made it widely popular in various fields of biomedical sciences [11], [12]. ML, a subset of AI, involves the design of algorithms and focuses on accurately predicting outcome variables. It evaluates the presumed interpretation based on a given dataset. Deep learning (DL), a component of ML, is another term associated with AI [13]. Computational algorithms based on AI analyze “training sets” using pattern recognition and data inputs to classify and predict models and patterns with higher precision and accuracy than traditional statistical modelling conducted by humans [14]. AI combines comprehensive datasets with computer-aided learning to emulate human intelligence, harnessing its problem-solving and decision-making capabilities [13]. By utilizing mathematical modeling and accurate predictions, AI can achieve tasks in biological sciences that were previously considered unattainable. It operates as a technology-driven system, employing a range of well-developed tools and networks to adopt a predictive approach. The medical field and the design of various drug delivery devices are among the numerous domains benefiting from AI’s applications [15], [16]. The proliferation of data from devices like wearable sensors, smartphones, and medical instruments has created a need for more accurate and efficient data analysis, which can be facilitated by DL algorithms [17]. Personalized medicine will become more prevalent in the future, although some physicians may hesitate to embrace rapid changes in established clinical practices [18].
However, it is important to acknowledge the limitations of AI/ML in pharmaceutical drug development. One such limitation is the potential for biases in analysis, which can arise from biased training data or algorithmic biases. Efforts must be made to address and mitigate these biases to ensure fair and unbiased results. Another challenge is the requirement for large datasets to train AI models effectively. Access to comprehensive and diverse datasets is crucial for accurate predictions and generalizability. Collaboration and data sharing among researchers and institutions can help overcome this challenge. Interpretability and transparency of AI models are also areas that require attention. Complex algorithms, often referred to as black boxes, can make it challenging to understand the reasoning behind their predictions. Developing methods to interpret and explain AI-driven results is essential for building trust and facilitating regulatory compliance [19].
Further exploration is needed to understand better how these models arrive at their predictions. Issues arise when incorporating new data and updating existing models, particularly in pharmaceutical research, where clinical trials and the introduction of new drugs generate vast amounts of data that must be processed using AI and ML models trained on existing datasets [20]. It demands that the process of results processing be changed through updates of AI tools. AI tools often showed limitations towards data variations. Many AI datasets are trained on extensive data repositories, potentially introducing biases towards average response values that may not align closely with the true average values crucial for accurate result analysis. This discrepancy can lead to erroneous predictions or substantial deviations from actual experimental readings [21], [22]. Ethical considerations surrounding the use of patient health data emphasize the importance of safety and security.
Ownership of data is another ethical concern, particularly in AI applications for drug development, where patient consent is often required for the use of their data. Such a process may induce conflicts among researchers, patients, and pharmaceutical industries for data ownership and utilization through AI tools [23], [24]. The effective integration of AI into drug development requires following a standard evaluation process, stringent protocols, and regulatory guidelines primarily related to animal welfare and patient safety. Accurate testing and validation are essential to enhance the reliability and accuracy of AI-generated predictions. Biological systems are often complex and dynamic, involving interconnected pathways, feedback loops, and intricate molecular interactions. Understanding and accounting for these complexities is vital for the successful application of AI in drug development. AI models are required to simplify the data generated from such complex biological systems during the drug development. The same AI often depends on the training data sets for pattern learning and then provide predictions of results. Such complexity requires challenging updates into AI models for data handling and unbiased results predication with minimum human interference [25], [26].
The emergent properties of biological systems give rise to system-level behaviors that pose challenges for accurate results prediction based solely on the properties of individual components. To address this, a comprehensive understanding of the workings, mechanisms, and processes of biological systems is necessary for incorporating them into AI models. This requires a workforce with clinical expertise that can effectively synchronize a statistical framework, AI models, biological responses observed through experiments, and predicted results derived from AI-trained datasets. By integrating these elements, we can enhance the accuracy and reliability of AI-driven predictions in the context of biological systems [27]. This review aims to explore AI applications in pharmaceutical research in drug development, drug delivery, drug discovery and biofabrication technology with the help of recent case studies and sophisticated AI tools used during the same. This review discussed several limitations of AI technology in the pharma sector, which can be addressed for better advancement in the field by the researchers. This review will help the ongoing research into AI develop gold-standard technological tools for the effective diagnosis and treatment of disease through significant contributions to pharmaceutical drug development and research.
Read more here
Amol D. Gholap, Md Jasim Uddin, Md. Faiyazuddin, Abdelwahab Omri, S. Gowri, Mohammad Khalid, Advances in Artificial Intelligence in Drug Delivery and Development: A Comprehensive Review, Computers in Biology and Medicine, 2024, 108702, ISSN 0010-4825, https://doi.org/10.1016/j.compbiomed.2024.108702.
Read more interessting articles on “Artificial Intelligence“ here:
- Artificial intelligence generates novel 3D printing formulations
- The Role of Artificial Intelligence in Generating Original Scientific Research
- Artificial Intelligence in Pharmaceutical Technology and Drug Delivery Design
