Interpretable artificial neural networks for retrospective QbD of pharmaceutical tablet manufacturing based on a pilot-scale developmental dataset
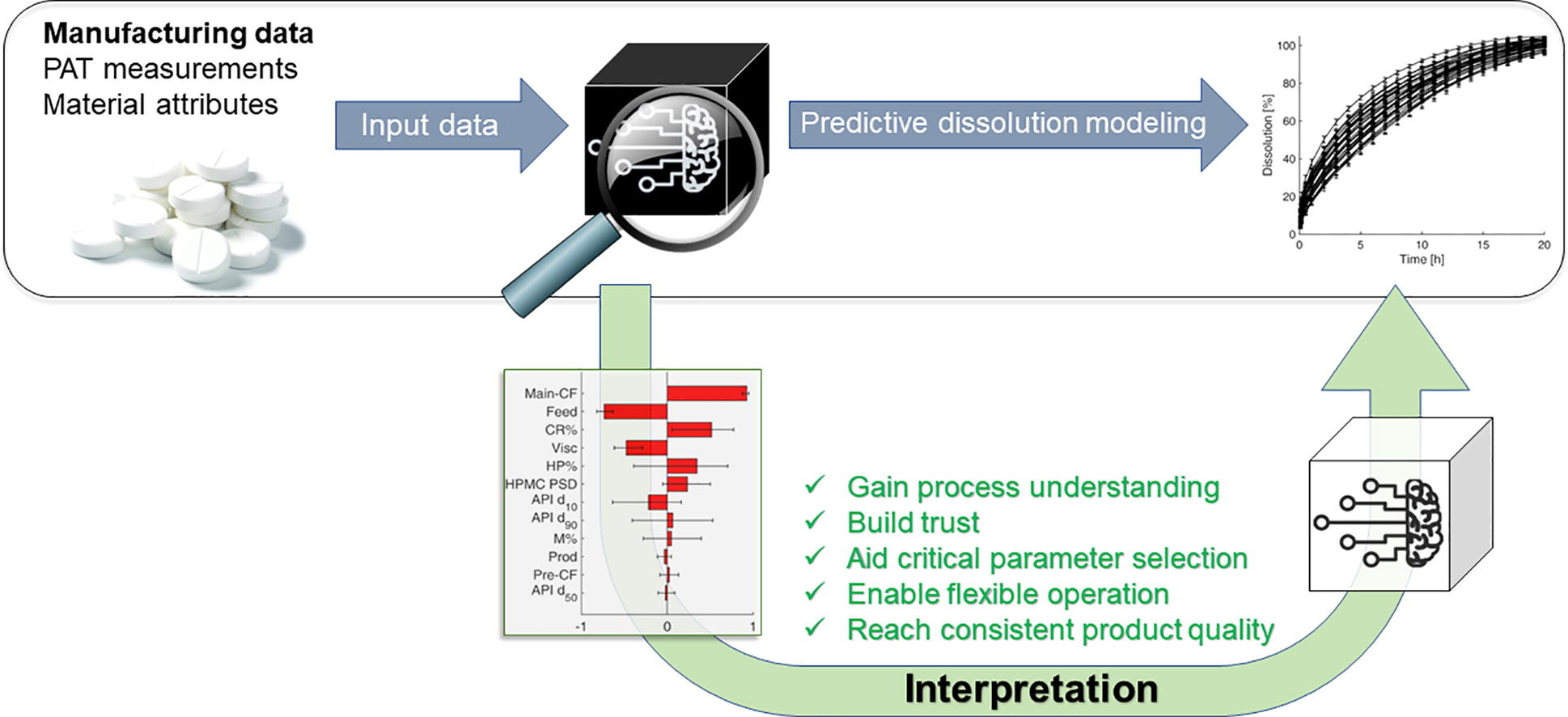
As the pharmaceutical industry increasingly adopts the Pharma 4.0. concept, there is a growing need to effectively predict the product quality based on manufacturing or in-process data. Although artificial neural networks (ANNs) have emerged as powerful tools in data-rich environments, their implementation in pharmaceutical manufacturing is hindered by their black-box nature. In this work, ANNs were developed and interpreted to demonstrate their applicability to increase process understanding by retrospective analysis of developmental or manufacturing data.
The in vitro dissolution and hardness of extended-release, directly compressed tablets were predicted from manufacturing and spectroscopic data of pilot-scale development. The ANNs using material attributes and operational parameters provided better results than using NIR or Raman spectra as predictors. ANNs were interpreted by sensitivity analysis, helping to identify the root cause of the batch-to-batch variability, e.g., the variability in particle size, grade, or substitution of the hydroxypropyl methylcellulose excipient.
An ANN-based control strategy was also successfully utilized to mitigate the batch-to-batch variability by flexibly operating the tableting process. The presented methodology can be adapted to arbitrary data-rich manufacturing steps from active substance synthesis to formulation to predict the quality from manufacturing or development data and gain process understanding and consistent product quality.
Download the full article as PDF here Interpretable artificial neural networks for retrospective QbD of pharmaceutical tablet manufacturing based on a pilot-scale developmental dataset
or read it here
Materials
A controlled-release tablet formulation was studied, containing the API in two different doses, silicified microcrystalline cellulose (PROSOLV® SMCC HD 90, JRS PHARMA GmbH & Co. KG) and lactose monohydrate (FlowLac® 100, Meggle, MEGGLE GmbH & Co. KG) as fillers, and magnesium stearate (Faci Asia Pacific Pte Ltd.) as a lubricant. Furthermore, 25 % w/w hydroxypropyl methylcellulose (HPMC) (METHOCEL™ K4M, DuPont) was used in controlled release (‘CR’) and direct compression grade (‘DC’) to form a hydrophilic gel layer, hence ensuring an extended release. The used K4M grade is a medium-molecular weight HPMC with approx. 4000 cPs viscosity (2% solution in water). Its DC and CR grades are chemically identical, with the same target viscosities and degrees of methyl and hydroxypropyl substitution (although batch-to-batch variabilities might occur due to the manufacturing). However, the DC grade has less fines and more spherical morphology to obtain a better powder flow rate, better processability and reproducibility in tablet weight and content uniformity. The exact composition of the drug formulation and the name and characteristics of the active pharmaceutical ingredient (API) are confidential.
Brigitta Nagy, Ágnes Szabados-Nacsa, Gergő Fülöp, Anikó Turák Nagyné, Dorián László Galata, Attila Farkas, Lilla Alexandra Mészáros, Zsombor Kristóf Nagy, György Marosi, Interpretable artificial neural networks for retrospective QbD of pharmaceutical tablet manufacturing based on a pilot-scale developmental dataset, International Journal of Pharmaceutics, 2023, 122620, ISSN 0378-5173, https://doi.org/10.1016/j.ijpharm.2023.122620.
Remember World Cancer Day 4th February 2023:
(For more information click on the picture)