Implementation of near-infrared spectroscopy and convolutional neural networks for predicting particle size distribution in fluidized bed granulation
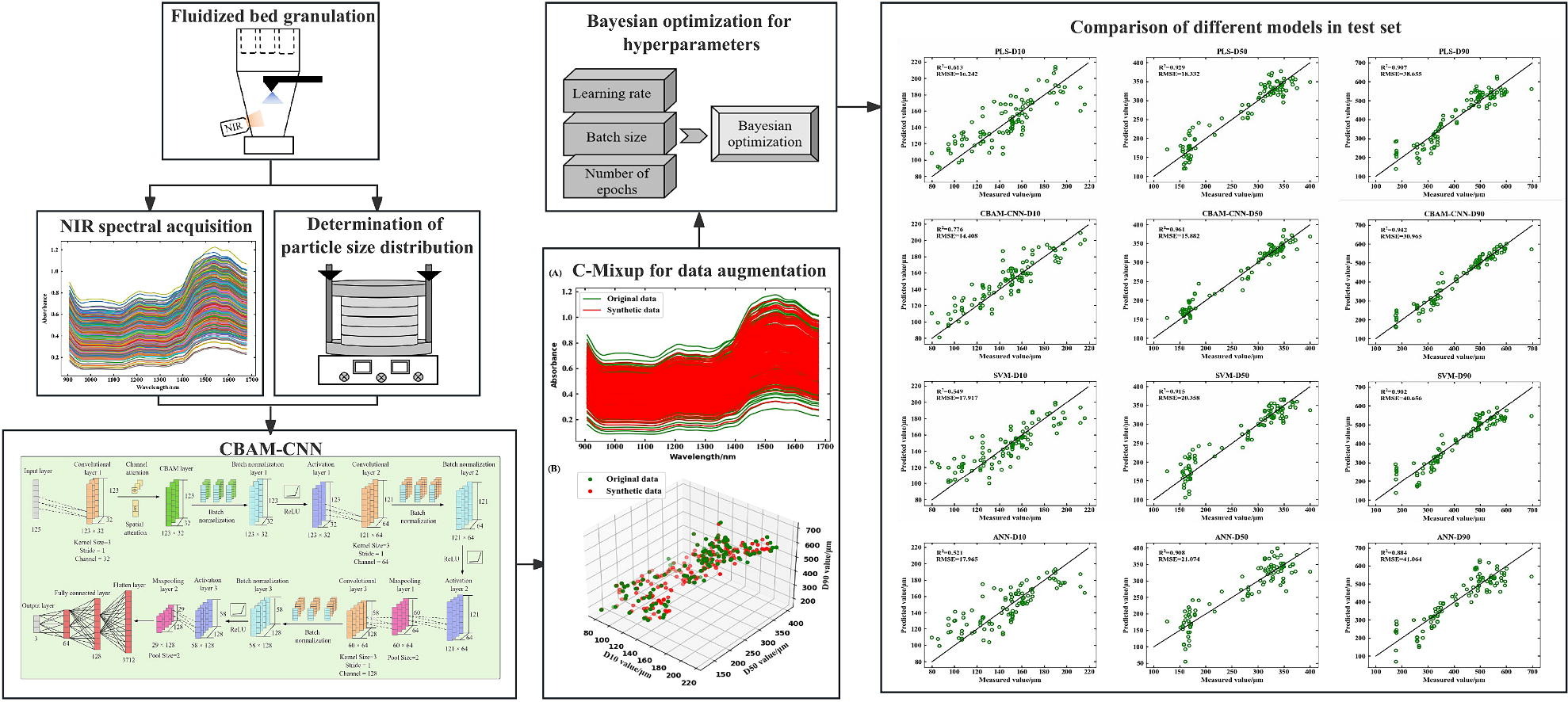
Monitoring the particle size distribution (PSD) is crucial for controlling product quality during fluidized bed granulation. This paper proposed a rapid analytical method that quantifies the D10, D50, and D90 values using a Convolutional Block Attention Module-Convolutional Neural Network (CBAM-CNN) framework tailored for deep learning with near-infrared (NIR) spectroscopy. This innovative framework, which fuses CBAM with CNN, excels at extracting intricate features while prioritizing crucial ones, thereby facilitating the creation of a robust multi-output regression model. To expand the training dataset, we incorporated the C-Mixup algorithm, ensuring that the deep learning model was trained comprehensively.
Highlights
- The first time using CBAM-CNN to predict particle size distribution during fluidized bed granulation.
- The CBAM-CNN model was optimized by introducing Bayesian optimization and C-Mixup algorithms.
- The performance of CBAM-CNN was compared to other classic models.
- The modeling convenience of CBAM-CNN was evaluated.
Additionally, the Bayesian optimization algorithm was introduced to optimize the hyperparameters, improving the prediction performance of the deep learning model. Compared with the commonly used Partial Least Squares (PLS), Support Vector Machine (SVM), and Artificial Neural Network (ANN) models, the CBAM-CNN model yielded higher prediction accuracy. Furthermore, the CBAM-CNN model avoided spectral preprocessing, preserved the spectral information to the maximum extent, and returned multiple predicted values at one time without degrading the prediction accuracy. Therefore, the CBAM-CNN model showed better prediction performance and modeling convenience for analyzing PSD values in fluidized bed granulation.
Read more here
Materials
In one batch, the granulation material (approximately 1600 g, 2:1 w/w) included lactose monohydrate (Armor Pharma ™ 80 Mesh, Amor, France) and microcrystalline cellulose (SH-CG1, 50 μm, Sunhere, China). The binder was a 10 % (w/w) aqueous solution of hydroxypropyl methylcellulose (SH-E3, Sunhere, China)
Cheng Peng, Liang Zhong, Lele Gao, Lian Li, Lei Nie, Aoli Wu, Ruiqi Huang, Weilu Tian, Wenping Yin, Hui Wang, Qiyi Miao, Yunshi Zhang, Hengchang Zang, Implementation of near-infrared spectroscopy and convolutional neural networks for predicting particle size distribution in fluidized bed granulation, International Journal of Pharmaceutics,
2024, 124001, ISSN 0378-5173, https://doi.org/10.1016/j.ijpharm.2024.124001.
Read also our introduction article on Binders here:
