Advanced process automation of a pharmaceutical continuous wet granulation line: Perspectives on the application of a model predictive control from solid feeders to dryer

Abstract
Pharmaceutical continuous manufacturing provides the appropriate tools (e.g. the understanding of process dynamics and appropriate and adaptable control strategy) in order to deal with Quality-by-Design expectations and even to the future smart manufacturing described by Quality-by-Control. Those tools form part of the given framework of the regulatory agencies led by an effective quality risk management. Soft sensors and control algorithms such as model predictive control are stepping stones for more agile processes and increased robustness by keeping the quality attributes of the final drug product in their acceptable ranges and by mitigating undesired events. The implementation of a model predictive control (MPC) system on a pharmaceutical continuous manufacturing plant for the wet granulation process is described.
The control objectives and strategy are presented as well as the selected variables, the process dynamics identification, the MPC performance and its specific tuning where a commercial software has been used, setting the framework of this study. MPCs have been applied successfully on two pharmaceutical drug products (Diclofenac and Paracetamol): an accurate control of the API content and of the LOD was achieved in order to produce a constant quality of tablets on both drug products. In addition, some of the process parameters have been identified as mandatory to be step tested for each change of drug product, leading to a simplified MPC implementation.
Introduction
As an emerging technology for pharmaceutical manufacturing, continuous manufacturing is being carefully studied, seeking for acceptable quality assurance. Frameworks (e.g. ICH Q13 [1], FDA draft guidance [2]) and first applications in industries [3] are emerging. Continuous manufacturing considers time as a new dimension, which requires understanding and inclusion of the process dynamics in the development of this new technology. Pharmaceutical continuous manufacturing is seeking for an appropriate and relevant control strategy [2] that should take into consideration the following points: the input material control to guarantee the adequate raw material quality and feeding consistency; the material transformation in response to the critical process parameters (CPP); the process monitoring in order to gather real time information on process parameters and quality attributes and in order to detect deviations and disturbances of the process; and accurate material diversion in case of out of specification (OOS) materials being produced (e.g. during transient phases or incidents) [3]. The material diversion relies on the material flow characterization by means of residence time distribution (RTD) [3,4]. Pharmaceutical continuous manufacturing is prompted to introduce an automated control system that adheres to the control strategy. Soft sensors and automated feed-back control can be helpful to keep the quality attributes as close as possible to their references and to mitigate the undesired events [3,5].
Pharmaceutical continuous manufacturing provides the framework to investigate proper ways to control the process variables in order to deliver high-quality pharmaceutical products. The appropriate control system is aimed to take corrective actions considering disturbances and uncertainties to move the product quality attributes toward desirable values. The literature describes three kind of control strategies [3,6,7] as shown in Fig. 1:
-
Quality-by-Testing (QbT), where the quality attributes of the product are tested at the end of each process step;
-
Quality-by-Design (QbD), where quality is built into the product while the critical quality attributes (CQA) and the critical process parameters (CPP) are identified and ranked [3,4,6,7];
-
Quality-by-Control (QbC), where an efficient and active control of the CPPs to achieve targeted CQAs is built into the process [3,6];
Continuous manufacturing provides the framework for QbD monitoring of the product’s quality attributes and for QbC, which would lead to smart manufacturing or Industry 4.0 [9,10].
Due to the high complexity of the pharmaceutical process (process constraints, multiple inputs and outputs), a proposed modern and promising control technique in pharmaceutical continuous manufacturing is model predictive control (MPC). Process modeling can help for both the risk assessment process and the evaluation of risk mitigation strategies, respectively through sensitivity and feasibility analyses [3,4].
MPC is a class of control algorithms; at each time point, it makes predictions about future plant outputs from process models [3,11]. Historically, MPC is an example of predictive control, widely used in highly dynamic situations such as autopilots [12], storage disk read/write heads [13,14] and weapons aiming [15] where predicting a short time into the future allows improved accuracy and speed of response. Much of modern control practice was developed during and after the second world war for military purposes. MPC-based control strategies have been used also in the petrochemical industries for optimized oil and gas production [3,16,17]. MPC control strategies are starting to be used in pharmaceutical continuous manufacturing either through simulation studies or real implementation in pharmaceutical plants [3]: from the control of one single operation unit such as the tablet press [3,5,6,[18], [19], [20]] to the control of an end-to-end continuous manufacturing pilot plant (from chemical synthesis to melt extrusion and tableting) [3,[21], [22], [23]].
A previous study [3] showed the MPC mode of action and a simplification of its control algorithm. The MPC principle is to solve a control problem within a finite prediction horizon and based on kinetic models describing the plant. These process models can be defined by three parameters: the dead time, the rising time (first order time constant FOTC representing 63.2% of the trend area) and the process gain. The cost function, which calculates the deviation from system states and from set points as a weighted sum of errors, should be the smallest possible. The optimal actuating signal is implemented in the next time step while the whole optimization problem is calculated again as the horizon window moves [3,[24], [25], [26]].
In this publication, the implementation of the MPC control system on a pharmaceutical continuous manufacturing plant for the wet granulation route is developed on two drug products by means of a commercial software from Emerson Automation Solutions. The control objectives and strategy are presented as well as the selected variables, the identification of the models used in the presented MPC, the MPC performance and its specific tuning with Diclofenac formulation as drug product. Then a second drug product has been tested for models identification and MPC performance in order to identify the process parameters that are mandatory to test for each change of drug product.
Read more here
Materials
Two drug products have been tested during this: Diclofenac formulation and Paracetamol formulation. Diclofenac Sodium (Acros Organics, Geel, Belgium) was used as active pharmaceutical ingredient (API). The standard Diclofenac formulation contained 25% diclofenac sodium, 0.5% aerosil 200 PH, 7.2% sodium starch-glycolate, 4.8% sodium stearyl fumarate, 3.9% Hypromellose (Cellulose-HP-M 603), 29.3% calcium hydrogen-phosphate anhydrous and 29.3% microcrystalline cellulose PH102
Morgane Jelsch, Yves Roggo, Mark Brewer, Zsolt-Adam Géczi, Philipp Heger, Peter Kleinebudde, Markus Krumme,
Advanced process automation of a pharmaceutical continuous wet granulation line: Perspectives on the application of a model predictive control from solid feeders to dryer, Powder Technology, 2023, 118936, ISSN 0032-5910, https://doi.org/10.1016/j.powtec.2023.118936.
Read more on Introduction to Sodium Stearyl Fumarate as a pharmaceutical excipient here:
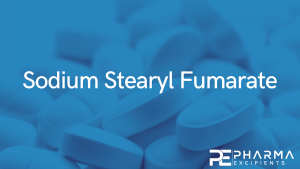